Evaluating the Quality of Clinical Documentation Using Maxim AI
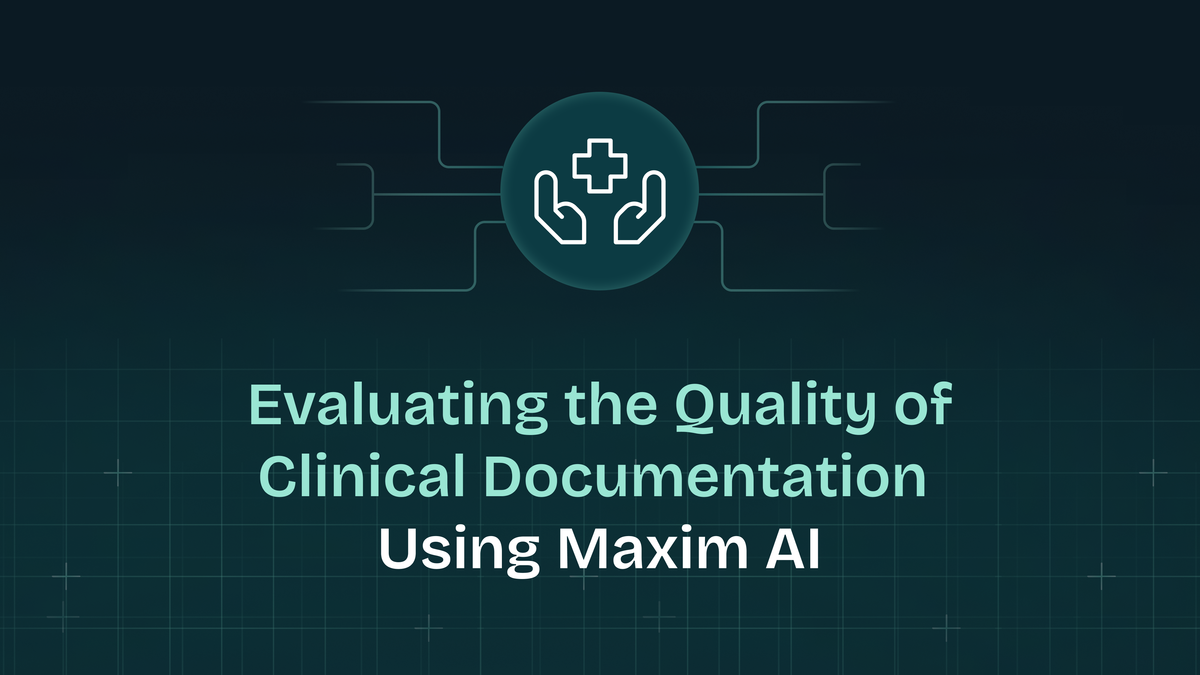
Medical note-taking and scribing assistants have eased the administrative burden on clinicians by ambiently generating clinical notes, allowing them to spend less time documenting and more time with patients.
In high-stakes clinical environments, it is essential to generate clear, accurate, and safe notes based on multi-turn interactions between clinicians and patients, ensuring reliable data is passed in EHRs.
In this blog, we'll prototype a medical assistant (in Maxim's prompt playground) that takes a conversation between doctor and patient as input and generates a structured clinical documentation. We'll focus on evaluating the quality of generated notes using Maxim's built-in and custom evals.
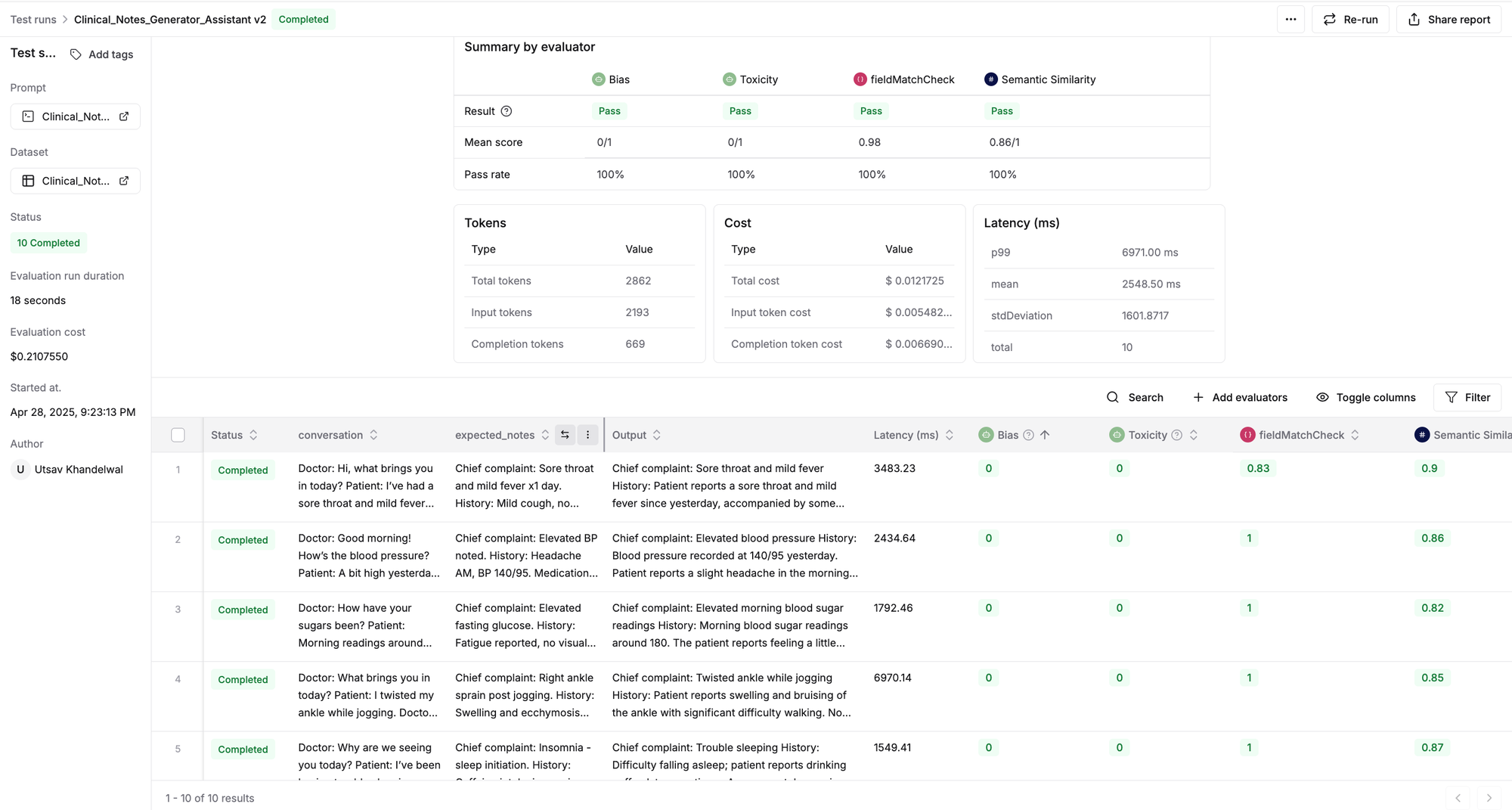
Step 1: Create a prompt experiment in Maxim
We'll define the prompt for our assistant using Maxim's no-code UI and use it to generate clinical notes with an LLM of choice.
- Create prompt: Head to "Single prompts" in the "Evaluate" section and click "+" to create a new prompt. Let's name this:
Clinical_Notes_Generator_Assistant
- Add system message: We'll use the following prompt to guide our scribing assistant to create clinical notes based on the conversation passed to it as input. Add this to the system message:
You are a clinical documentation assistant for healthcare professionals.
Your job is to read a multi-turn conversation between a doctor and a patient and generate a structured clinical note based on the interaction.
Follow these rules carefully:
- Do NOT include any unnecessary commentary or disclaimers.
- The note should be clear, concise, and use standard medical terminology.
- Maintain an objective and professional tone.
The clinical note should follow this structure:
Chief complaint: [Main reason the patient came in]
History: [Symptoms, duration, context, relevant negatives]
Medications: [Current medications if mentioned]
Allergies: [Any known allergies]
Assessment: [Doctor’s impression or working diagnosis]
Plan: [Next steps – investigations, prescriptions, follow-ups]
Only include fields that are mentioned above.
3. Select model: Choose from the wide range of models available on Maxim. We'll use GPT-4o to generate clinical notes for this example. For factual generation, keep the model temperature low (recommended range- .2 to .3)
- To test the output, we'll pass the following user message to the assistant we prototyped. This message contains a multi-turn interaction between the clinician and the patient.
Doctor: Hi, what brings you in today?
Patient: I've had a sore throat and mild fever since yesterday.
Doctor: Any cough or difficulty swallowing?
Patient: Some coughing, but no trouble swallowing.
Doctor: Any known allergies or meds you're on?
Patient: Just cetirizine occasionally.
We'll now create a golden dataset, which is a collection of doctor-patient interactions and corresponding expected notes. We'll use these expected notes to evaluate the performance and quality of the notes generated by our assistant.
Step 2: Create a dataset on Maxim
Download this sample dataset as CSV. This contains 10 real-world doctor-patient conversations (that we'll pass as input to our assistant) and their expected clinical notes.
- To upload the dataset to Maxim, go to the "Library" section and select "Datasets".
- Click the "+" button and upload a CSV file as a dataset.
- Name your dataset:
Clinical_Notes_Assistant_Dataset
and ensure "First row is header" is checked
- Map the columns in the following manner:
- Set
conversation
as "Input" type, since these conversations will be the input to our assistant for clinical note generation. - Set
expected_notes
as "Expected Output" type, since this is the reference for comparison of generated clinical notes.
- Set
- Click "Add to dataset" and the golden dataset for your evaluations is ready for use.
Step 3: Evaluating the assistant
Now we'll evaluate the performance of our assistant and the quality of generated clinical documentation.
- Go to the Prompt Playground and click "Test" in the top right corner.
- Select the dataset, i.e.
Clinical_Notes_Assistant_Dataset
. - Selecting the evaluators:
- Maxim provides a comprehensive list of AI (LLM-as-a-judge), programmatic, and statistical evaluators. Additionally, you can add more evaluators from Maxim's evaluator store by clicking "Browse evaluator store".
- To evaluate the clinical documentation, we'll use the following evals available on Maxim.
Evaluator | Type | Purpose |
---|---|---|
Semantic similarity | Statistical | This assessment validates that generated output is semantically similar to expected output. |
Bias | LLM-as-a-judge | This Metric determines whether output contains gender, racial, political, or geographical bias |
Toxicity | LLM-as-a-judge | This metric evaluates toxicness in your LLM outputs. |
- We’ll also create a custom programmatic evaluator that will check if the defined fields, such as "Chief complaint", "History", "Medications", that are present in the expected output, are present in the output as well.
- Head to the Evaluators page and click "+" to create a custom Programmatic Evaluator.
- Enter the following code in the IDE, and let’s name this evaluator
fieldMatchCheck
. Click "Save".
// Programming language used: JavaScript
function validate(output, expectedOutput) {
const searchStrings = ["chief complaint", "history", "medications", "assessment", "plan", "allergies"];
let matchCountOutput = 0;
let matchCountExpectedOutput = 0;
for (const searchTerm of searchStrings) {
if (expectedOutput.toLowerCase().includes(searchTerm)) {
matchCountExpectedOutput += 1;
if (output.toLowerCase().includes(searchTerm)) {
matchCountOutput += 1;
}
}
}
return (matchCountOutput / matchCountExpectedOutput);
}
- Maxim supports creating programmatic evaluators in Python and JavaScript.
This custom eval will be available for us to use while running an evaluation on our assistant
- Now, trigger the test run. For each of the conversations in our dataset, our model will generate clinical notes and evaluate the quality based on the metrics we've defined through our evaluators.
- Upon completion of the test run, you’ll see a detailed report of our assistant's performance.
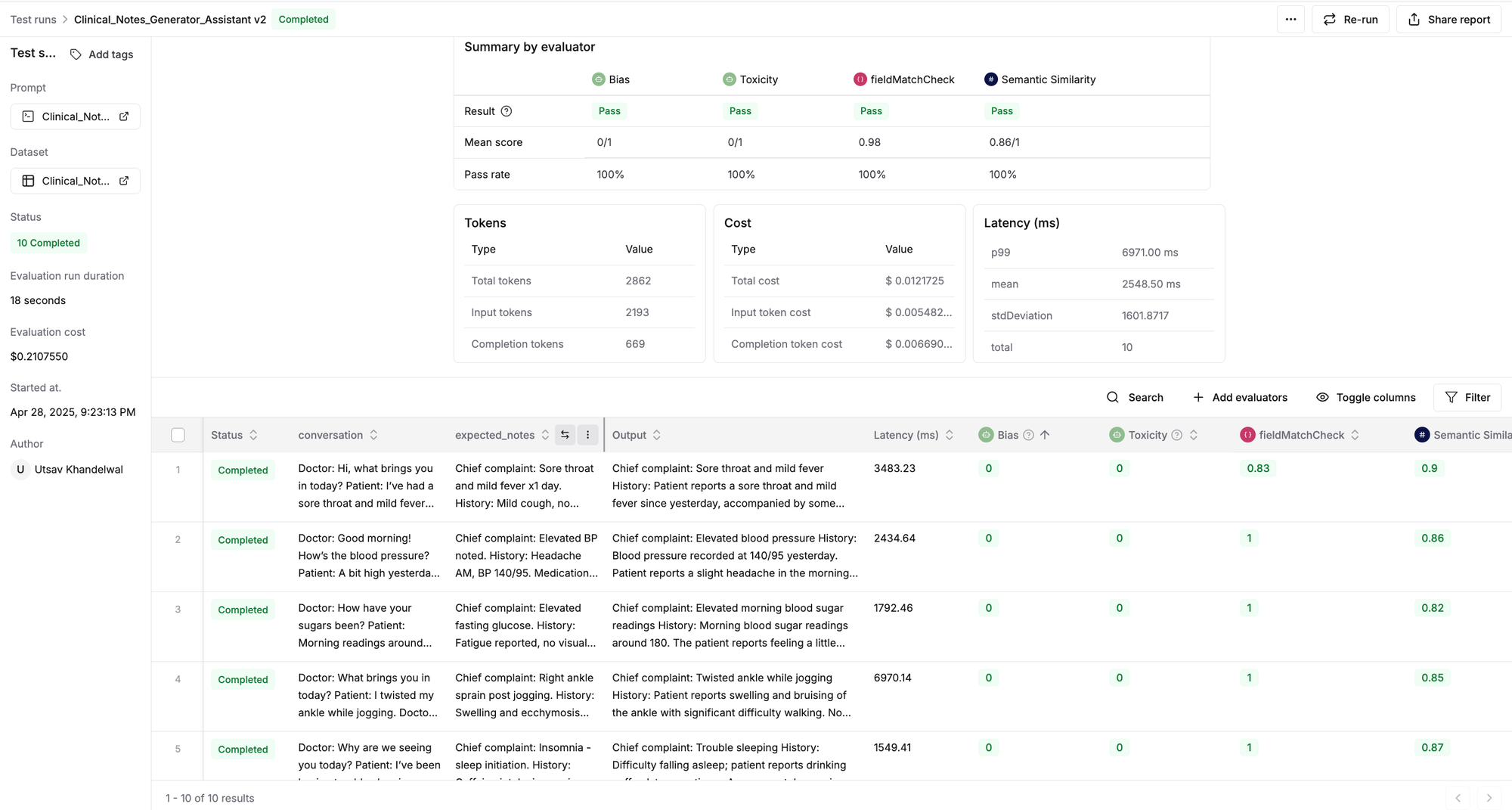
Check out the dynamic evaluation report generated for our assistant. You can click on any row to inspect the input conversation, the generated clinical note, or the evaluator's scores and reasoning.
Conclusion
With Maxim’s no-code prompt playground, setting up a basic prompt and uploading a reference dataset takes just minutes. The true value, however, lies in how you measure and maintain the quality of every generated note.
- Diverse evaluator suite: Run each batch of notes through checks for clarity, factual completeness, bias, toxicity, and alignment with expected outputs.
- Actionable feedback loop: Use evaluator scores and any human review notes to refine prompts, adjust dataset examples, or tweak model settings.
- Scenario coverage: Gradually expand tests across different specialties, patient demographics, and edge-case conversations to uncover weaknesses early.
By centering your workflow on continuous evaluation and rigorous quality assurance, you can turn your LLM outputs into reliable clinical documentation. Clinicians get clear, accurate notes in their EHRs, and you gain confidence that every note meets the highest standards for patient safety and care. Get started with Maxim!