The Era of Experience: Vision for the Next Frontier in AI
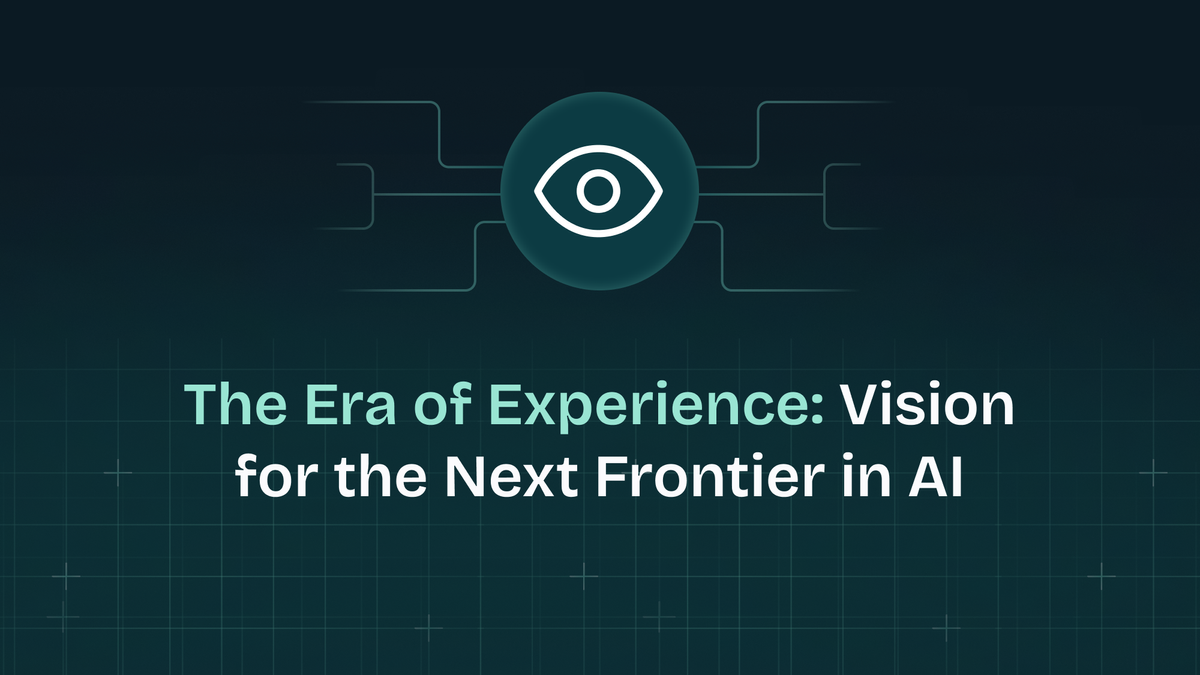
In this recent paper, The Era of Experience, David Silver and Richard Sutton articulate a vision for artificial intelligence where there is a shift from reliance on static, human-generated data to dynamic, self-generated experiential learning. This paradigm aims to propel current models beyond their limitations, developing systems capable of continuous learning and adaptation through interaction with their environments.
From Human Data to Experiential Learning
The Simulation Era
Early AI systems, such as DeepMind's AlphaGo, achieved remarkable feats by training in simulated environments. These systems utilized reinforcement learning (RL) to master complex tasks, such as playing Go, by interacting with digital simulations through self-play that provided clear rules and feedback through rewards like winning the game.
The Era of Human Data
The advent of large language models (LLMs) marked a significant shift, where models began to learn from a vast corpora of human-generated text. Models like ChatGPT demonstrate impressive abilities in language understanding and generation by training on diverse datasets encompassing books, articles, and online content.
However, this approach has inherent limitations. The reliance on existing human data constrains the potential for models to surpass human-level performance, especially in domains where data is scarce or non-existent. Moreover, the finite nature of high-quality human data poses a bottleneck for continued progress.
The Emergence of the Era of Experience
The authors propose that the next significant leap in AI will be driven by systems that learn through their own experiences. By interacting with environments, making decisions, and observing outcomes, AI agents can generate their own data, leading to continuous and autonomous learning.
Case Study: AlphaProof
AlphaProof was along the lines of this paradigm shift. Initially trained on approximately 100,000 formal proofs, AlphaProof employed RL to interact with a formal proving system, generating over 100 million additional proofs. This self-generated data enabled AlphaProof to achieve a medal in the International Mathematical Olympiad, surpassing the capabilities of models trained solely on human data.
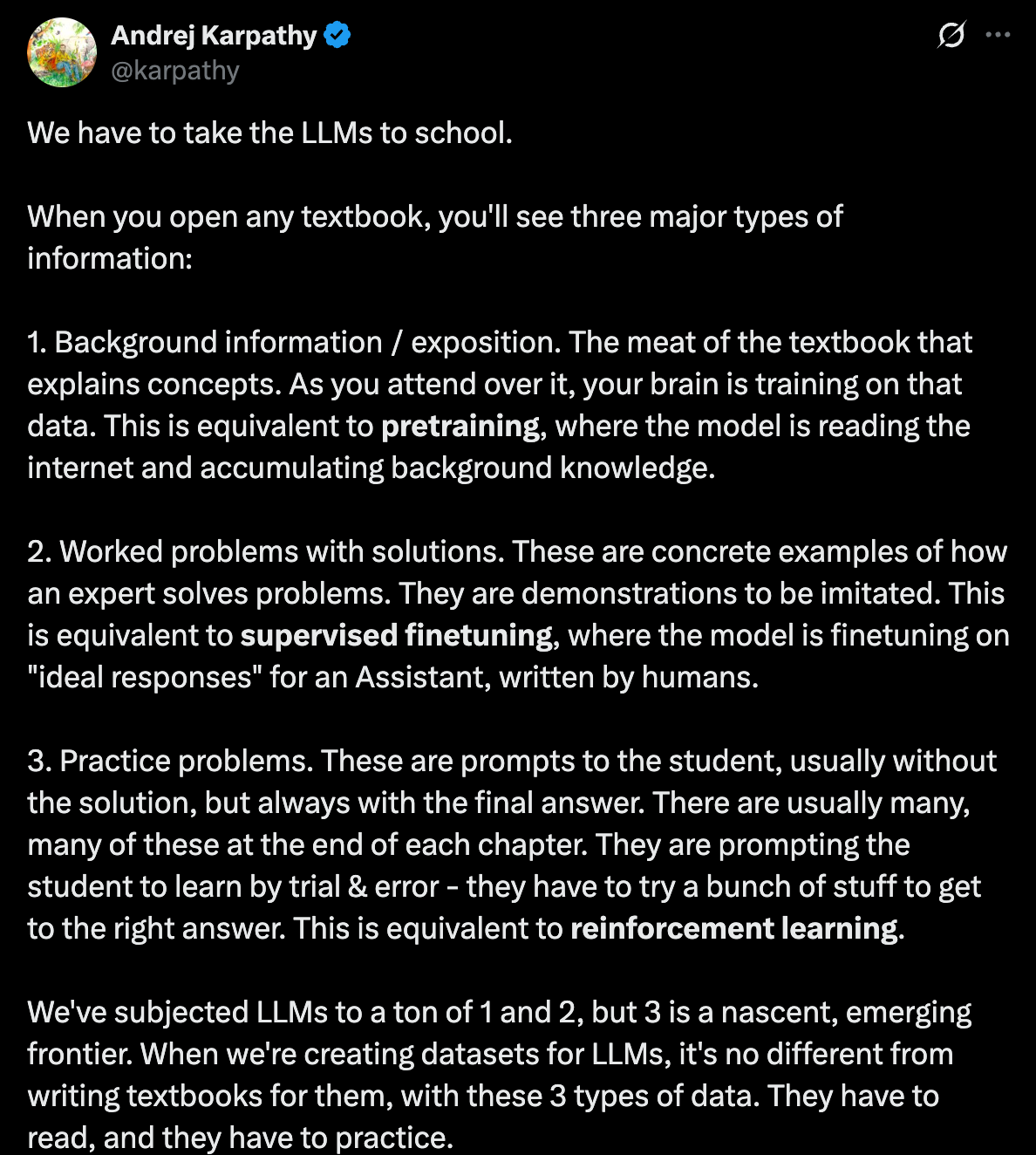
Key Characteristics of Experiential AI
The transition to experiential learning entails several fundamental changes in how AI systems operate:
1. Continuous Learning from Streams of Experience
Unlike traditional models that learn from static datasets, experiential AI agents engage in ongoing interactions with their environments, allowing for continuous learning and adaptation.
2. Rich Environmental Grounding
Experiential agents perceive and act within complex environments, grounding their learning in rich sensory inputs and contextual information beyond textual data.
3. Intrinsic Reward Mechanisms
These agents derive rewards from their interactions with the environment, enabling them to develop behaviors and strategies that are not explicitly programmed but emerge from the pursuit of goals within the environment like humans do.
4. Planning and Reasoning Based on Experience
Experiential AI systems utilize their accumulated experiences to inform planning and decision-making, allowing for more sophisticated and context-aware behaviors.
For example, a science agent with a goal to reduce global warming might use a reward based on empirical observations of carbon dioxide levels, while a goal to discover a stronger material might be grounded in a combination of measurements from a materials simulator, such as tensile strength or Young’s modulus.
Implications and Future Directions
The shift towards experiential learning has profound implications for the development of AI:
- Enhanced Generalization: By learning from diverse experiences, AI systems can develop more generalized and robust models, capable of adapting to novel situations.
- Reduced Dependence on Human Data: Experiential learning mitigates the limitations imposed by the availability and quality of human-generated data, enabling AI to explore and learn beyond human knowledge.
- Accelerated Innovation: As AI systems autonomously explore and learn, they can potentially uncover novel insights and solutions, driving innovation across various domains.
However, this approach also presents challenges, including ensuring the safety and alignment of AI behaviors, managing the complexity of real-world environments, and developing algorithms capable of effectively leveraging experiential data.
Conclusion
The "Era of Experience" brings an interesting perspective and a transformative phase in artificial intelligence, where systems learn and evolve through their own interactions with the world. By embracing experiential learning, AI can overcome the constraints of human data, achieving greater autonomy, adaptability, and intelligence. As we stand at the threshold of this new era, the insights of Silver and Sutton provide a compelling roadmap for the future of AI development.
For more information, please access the full paper here: The Era of Experience.